By John Ezekowitz
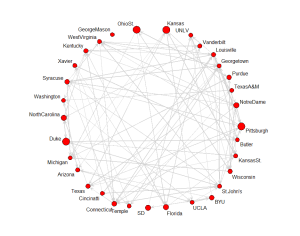
Each March, college basketball fans looking for help in filling out their NCAA Tournament brackets can go one of two ways: the quantitative, algorithmic route used effectively by sites like Ken Pomeroy and TeamRankings, or the “expert opinion” route, which uses more qualitative measures to value teams. Both have their strengths, but also leave things to be desired. Science and experience have shown again and again that we are often led astray by simply following qualitative judgments entirely. Likewise, relying completely on quantitative measures ignores potentially important unquantified traits like confidence and preparedness.
More generally, almost all prediction methods make the dubious assumption that NCAA Tournament games are the same as regular season games. That does not seem to hold true. NCAA Tournament games are played in bigger arenas, under brighter media spotlights, and with higher stakes than almost any regular season game.
I believe I have come up with a third way of predicting the NCAA Tournament that rests on the opposite assumption: Tournament games are different, and should be predicted differently. Using network analysis tools to marry the qualitative and quantitative prediction models, I attempt to quantify teams’ confidence and preparedness for the Tournament. My model performed better out of sample in predicting the 2010 NCAA Tournament than other algorithmic models, correctly predicting 44 of the 63 games and two Final Four teams (West Virginia and Duke).
The model is based on two facets: team strength controls, and the network analysis component. It is vital to have measures of regular season strength as they will of course be predictive of NCAA success. As such, I used Ken Pomeroy’s Pythagorean rankings (fully explained here), his Strength of Schedule measure, and his measure of a teams’ Consistency. The first two are standard and intuitive, while the last one is more interesting. Consistency is “the standard deviation of the scoring difference of games for a team.” Teams with lower numbers tend to be more consistent, producing similar margins in their games. Consistency can be used as a measure of a team’s in-season strength because under the assumption that the teams being analyzed are good teams, it represents a measure of variance of performance, a team’s ceiling (Mike James has done some awesome work on this). This seems a fair assumption, as there are very few “consistently bad” teams in the NCAA Tournament.
All of these factors are statistics that measure regular season team success and strength. But as mentioned before, the NCAA Tournament is a different arena, with much brighter lights and perhaps different determinants of success. Analyzing the NCAA Tournament network of games played between NCAA teams can provide insights into how teams within the Tournament are affected by their history of interactions with top-tier teams. More subtle psychological factors, like confidence and performance under pressure, can be measured via a network approach.
Imagine this hypothetical: Team A has played a very tough schedule, facing thirteen teams that are in the NCAA Tournament field and beating seven of them. Team B has played an easier schedule, only having played threeTournament teams, but it has defeated all of them. Through the vagaries of the season, and through games against non-tournament opponents, Team A and Team B have very similar Pythag ratings and consistency metrics. But Team A and Team B inhabit very different parts of the NCAA Tournament network. General statistical models might predict Team A and Team B to have equal tournament success, but we might hypothesize that Team A will do better because they have confidence from having played and defeated quite a few NCAA Tournament teams.
To quantify a team’s place in the network, I calculate a measure of weighted degree centrality. I call this variable Weighted Wins. Weighted Wins is the dot product of a team’s wins against other NCAA Tournament teams and the inverse of those teams’ seeds. Because the bracket is seeded by the committee from 1 to 16, seed acts as an exogenous proxy for quality of team. This weighting scheme gives more credit for defeating a 2 seed (1*1/2 Weighted Wins) than defeating a 15 seed (1*1/15 Weighted Wins), because defeating a 15 seed should be much easier than beating a 2 seed. For example, if Team B beat two 12 seeds and a 6 seed during the regular season, their WW would be (1/12 + 1/12 + 1/6) = 1/3, or .33. More Weighted Wins represents a better performance against NCAA Tournament teams during the regular season.
Another “intangible” variable often cited by experts as important for NCAA Tournament success is experience. To quantify experience, specifically experience in the NCAA Tournament, I used a dataset of minutes played at the individual player level for every year from 2007 until 2011 to create Returning Minutes Percentage for each team. As far as I know, this variable has not been calculated in the public domain before. Returning Minutes % for a team was then multiplied by the number of NCAA Tournament wins the team had in the previous year. Sensibly, this makes the experience term proportional to both past NCAA Tournament success and the percentage of players returning who contributed to that success.
Theoretically, NCAA Tournament experience and confidence should be interrelated, and as it turns out, an interaction term between Weighted Wins and the experience variable plays an important part in the final model.
To build the model, I used data from five NCAA Tournament seasons (2007-2011). This range was the largest I could do, as the data I used to calculate Returning Minute Percentage only went back that far (major thanks to TeamRankings for providing that data). To measure NCAA Tournament success, I used NCAA Tournament wins, which can range from 0 to 6. Because NCAA wins is a discrete variable, I used an ordered probit regression instead of a simple Ordinary Least Squares.
The results of this regression were stunning. I’m not going to include the full regression table here, but it is available upon request. Both Weighted Wins and NCAA Experience are significant predictors of NCAA Tournament success, controlling for Pythagorean Expectation and Consistency. The interaction term between Weighted Wins and NCAA Experience (literally multiplying the two together) is also significant at the 10 percent level, and an F-test of the interaction and the lower order terms is statistically significant (p value= 0.047). More importantly, the coefficient on the interaction term is positive, which shows that more Weighted Wins and more NCAA Tournament experience leads to more NCAA success, even when controlling for regular season team strength. This result confirms the hypothesis that network analysis yields additional predictive power above and beyond purely statistical measures of regular season success.
In order to test the strength of the model, I used pseudo-out-of-sample testing. That is, I took the 2010 NCAA Tournament teams out of the regression, ran it again, and then applied the new coefficients to the 2010 teams. Thus the model gave me a ranking of the teams in the 2010 Tournament, which I used to fill out a bracket and see how well it did. I compared my results to those predicted by TeamRankings, Ken Pomeroy, and the national consensus on ESPN.com. Here is what my predicted bracket looked like:
The model correctly predicted 44 games, and got two Final Four teams right. I scored the brackets using ESPN.com’s standard 1/2/4/8/16/32 scoring system (and the results from Audacity of Hoops ). As you can see from the table below, my Weighted Wins model had more points than Pomeroy’s rankings, the TeamRankings bracket, and the public consensus.
Needless to say, I was quite pleased by the out of sample test results.
This model strongly suggests that the assumption that NCAA Tournament games are the same as regular season games, and should be predicted as such, does not hold. Previously unquantified “intangibles” like preparednesss and confidence seem to be significant predictors of NCAA Tournament success. This model is also a huge success for the ability of network analysis techniques to quantify these traits and for the application of network analysis to predicting basketball games in general.
Of course, there are always concerns and ways to improve the model further. One obvious way would be to include margin of victory in Weighted Wins. This would improve the model’s accuracy if, and only if, beating a team by a larger margin increased the confidence or preparedness gained from that win. Another caveat is sample size. I was only able to use five years of NCAA Tournament data. That is enough data to make conclusions, but more data could improve the strength of the model. The nice thing is that the sample size will improve as future Tournaments are played.
Predicting NCAA Tournament success is a more subtle problem than simply identifying the “best team” from the regular season. The Tournament’s single-elimination format makes results far more random than most other playoff formats, which use multiple-game series. This means that ranking systems that do a very good job of classifying team strength over the course of the regular season may not be the best strategy for predicting postseason success.
This model, which to my knowledge has never been tried before, could lead to an entirely new method for quantifying the traits that breed NCAA Tournament success. Next March, fans looking for the best bracket prediction strategy may no longer have to choose between statistical rigor and expert opinion.
You can continue to backtest the system and see how it did 2005-2006.
Actually, I can’t because no one has returning minutes for teams in 2005 and 2006. Without that, the model breaks down.
How did this do in 2012 relative to Kenpoms?
Initial reactions (not having read the full paper yet, so I apologize if these are covered therein):
1) Great idea to use returningMinutes*NCAAwins as a factor. That makes perfect sense, and meshes well with conventional wisdom and some pseudo-scientific methods I’ve seen. I’d also be curious to see the effect of using NCAA games rather than NCAA wins, since losing in the first round might be valuable, just in terms of getting familiar with the experience.
2) Weighted Wins seems very dependent on the selection committee, who in theory should have no effect on a team’s performance/confidence. For example, the difference between beating a 1-seed and a 2-seed (which could be only 1 spot on the S-curve) is enormous. Beating [3,4,5] is worth more than beating [2] but less than beating [1].
3) If the Consistency term that you’re using is the same one that Pomeroy used to publish with his ratings, isn’t that NOT adjusted for opponent strength? If so, low consistency might actually mean that the team plays up and down to its opponents, which I would probably call inconsistent. Then again, playing up to your opponents could be a good sign, if you’re the underdog in a game.
4) I’m glad somebody found my Prediction System Bracket Challenge useful. 🙂 On that note, how would this model have done in this year’s tournament? I assume UConn would have been rated highly. But the Big East in general likely would have, and other than UConn, they didn’t do so well.
Your south bracket has some errors:
Texas A&M v. Utah State
and
Purdue v. Siena
are missing (KSU, Wisconsin, and Belmont shouldn’t be there)
Howdy! I realize this is kind of off-topic but I had to ask.
Does building a well-established blog like yours take a massive
amount work? I’m brand new to writing a blog however I do write in my diary on a daily basis. I’d like to start a blog so I will be able to share my experience and feelings online.
Please let me know if you have any kind of ideas or tips for brand new aspiring
bloggers. Appreciate it!
I’m not that much of a online reader to be honest but
your sites really nice, keep it up! I’ll go ahead and bookmark your website to come back later on. Cheers